THE 19TH AUSTRALASIAN DATA MINING CONFERENCE 2021 (AUSDM’21)
Brisbane, Australia, 14-15 December 2021
AusDM’21 will be Running Virtually due to COVID-19 Restrictions
Welcome to AusDM’21

- Paper submissions:
24 August7 Sept 2021 - Notification: 3 October 2021 2021
- Camera-ready: 17 October 2021
The Australasian Data Mining Conference has established itself as the premier Australasian meeting for both practitioners and researchers in data mining. It is devoted to the art and science of intelligent analysis of (usually big) data sets for meaningful (and previously unknown) insights. This conference will enable the sharing and learning of research and progress in the local context and new breakthroughs in data mining algorithms and their applications across all industries.
Since AusDM’02 the conference has showcased research in data mining, providing a forum for presenting and discussing the latest research and developments. Built on this tradition, AusDM’21 will facilitate the cross-disciplinary exchange of ideas, experience and potential research directions. Specifically, the conference seeks to showcase: Research Prototypes; Industry Case Studies; Practical Analytics Technology; and Research Student Projects. AusDM’21 will be a meeting place for pushing forward the frontiers of data mining in academia and industry.
Publication and Topics
We are calling for papers, both research and applications, and from both academia and industry, for publication and presentation at the conference. All papers will go through double-blind, peer–review by a panel of international experts. The AusDM’21 proceeding will be published by Springer-Verlag and become available immediately after the conference.
Please note that AusDM’21 requires that at least one author for each accepted paper register for the conference and present their work.
AusDM invites contributions addressing current research in data mining and knowledge discovery as well as experiences, novel applications and future challenges.
Topics of interest include, but are not restricted to
• Applications and Case Studies — Lessons and Experiences
• Big Data Analytics
• Biomedical and Health Data Mining
• Business Analytics
• Computational Aspects of Data Mining
• Data Integration, Matching and Linkage
• Data Mining in Education
• Data Mining in Security and Surveillance
• Data Preparation, Cleaning and Preprocessing
• Data Stream Mining
• Deep Learning
• Evaluation of Results and their Communication
• Implementations of Data Mining in Industry
• Integrating Domain Knowledge
• Link, Tree, Graph, Network and Process Mining
• Machine Learning
• Multimedia Data Mining
• New Data Mining Algorithms
• Professional Challenges in Data Mining
• Privacy-preserving Data Mining
• Spatial and Temporal Data Mining
• Text Mining
• Visual Analytics
• Web and Social Network Mining
Keynote Speakers
As is tradition for AusDM, we have lined up an excellent keynote speaker program. Each speaker is a well-known research and/or practitioner in data mining and related disciplines. The keynote program provides an opportunity to hear from some of the world’s leaders on what the technology offers and where it is heading.
Talk 1: Why Multilayer Networks are Needed for Complex Data Analysis
Abstract: We are on the cusp of holistically analyzing a variety of diverse data being collected in every walk of life. For this, current analytics and science are being extended (Big Data Analytics/Science) along with new approaches. This warrants developing and/or using new approaches – technological, scientific, and systems – in addition to building upon and integrating with the ones that have been developed so far.
After going through the mining/analysis landscape, we present the need for multilayer networks for complex data analysis. Although mining/analysis of data using graphs has been around for a while, it has come to the forefront due to Internet and social networks as well as its versatility to model complex data sets. In this talk, we argue that graph analysis techniques are extremely important and hence renewed attention is needed as the data sets become complex.
We first illustrate the elegance of multilayer networks for modeling by using a few well-known data sets. We show how multiple entity and multiple relationships in data can be modeled elegantly using MLNs as compared to other representations. Flexibility of analysis comes from modeling the data MLNs. Then we discuss the “decoupling-based” approach for computation efficiency and scalability. This “divide and conquer” approach composes computations (e.g., communities, hubs, and substructures) from individual layers to form loss-less computation for any combination of layers in a multilayer network. Finally, we present several diverse case studies to showcase the approach.
Speaker: Professor Sharma Chakravarthy, The University of Texas at Arlington, Arlington, Texas

Prof. Sharma Chakravarthy is an ACM Distinguished Scientist and Distinguished speaker. He is also an IEEE Senior Member. He is also a Fulbright specialist. He organized (General Co-Chair) the 13th international Conference on Distributed Event-Based Systems (DEBS 2013). He has spent several summers at the Rome Air Force Research Laboratory (AFRL) as a Faculty Fellow working in continuous query processing over fault-tolerant networks and video stream analysis.
Sharma Chakravarthy is Professor of Computer and Engineering Department at The University of Texas at Arlington, Texas. He established the Information Technology Laboratory at UT Arlington in Jan 2000 and currently heads it. Sharma Chakravarthy has also established the NSF funded, Distributed and Parallel Computing Cluster (DPCC@UTA) at UT Arlington in 2003. He is the recipient of the university-level “Creative Outstanding Researcher” award for 2003 and the department level senior outstanding researcher award in 2002.
He is well known for his work on stream data processing, semantic query optimization, multiple query optimization, active databases (HiPAC project at CCA and Sentinel project at the University of Florida, Gainesville), and more recently scalability issues in graph mining, social network analysis, and graph analysis of multilayered networks. His group at UTA is currently adapting map/reduce and other paradigms for scaling graph mining algorithms to very large graphs and for answering graph queries. He has applied machine learning techniques to rank answers, identify general- and topic-based experts in a Question-Answer (or Q-A) social network. His work on InfoSift – a classification system for text, email, and web – has used graph mining techniques.
His current research includes big data analysis using multi-layered networks, stream data processing for disparate domains (e.g., video analysis), scaling graph mining algorithms for analyzing very large social and other networks, active and real-time databases, distributed and heterogeneous databases, query optimization (single, multiple, logic-based, and graph), and multi-media databases. He has published over 200 papers/book chapters in refereed international journals and conference proceedings. He has supervised 15+ PhD theses and 90+ MS thesis. He has given tutorial on a number of database topics, such as graph mining, active, real-time, distributed, object-oriented, and heterogeneous databases in North America, Europe, and Asia. He is listed in Who’s Who Among South Asian Americans and Who’s Who Among America’s Teachers.
Prior to joining UTA, he was with the University of Florida, Gainesville. Prior to that, he worked as a Computer Scientist at the Computer Corporation of America (CCA) and as a Member, Technical Staff at Xerox Advanced Information Technology, Cambridge, MA.
Sharma Chakrvarthy received the B.E. degree in Electrical Engineering from the Indian Institute of Science, Bangalore and M.Tech from IIT Bombay, India. He worked at TIFR (Tata Institute of Fundamental Research), Bombay, India for a few years. He received M.S. and Ph.D degrees from the University of Maryland in College park in 1981 and 1985, respectively.
Talk 2: An introduction to semi-supervised learning and contrastive loss
Abstract: In the last couple of years there has been a surge of interest in “self-supervised learning” (SSL). This is where we train a deep learning model using labels that are naturally part of the input data, rather than requiring separate external labels. SSL allows us to train with fewer labels, which has opened up many new domains to deep learning. Although the idea goes back to 1989 (Jürgen Schmidhuber), recent results have been particularly impressive thanks to the use of Large Language Models, and also the recent development of “consistency loss” (as it is known in NLP, or “noise contrastive estimation” in computer vision). This talk will cover the history of these ideas, the key ideas behind them, look at different approaches to implementing them, and discuss their results.
Speaker: Jeremy Howard, CSIRO, fast.ai
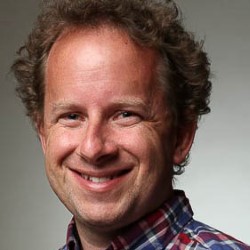
Jeremy Howard is a data scientist, researcher, developer, educator, and entrepreneur. Jeremy is a founding researcher at fast.ai, a research institute dedicated to making deep learning more accessible. He is also a Distinguished Research Scientist at the University of San Francisco, the chair of WAMRI, and is Chief Scientist at platform.ai.
Previously, Jeremy was the founding CEO Enlitic, which was the first company to apply deep learning to medicine, and was selected as one of the world’s top 50 smartest companies by MIT Tech Review two years running. He was the President and Chief Scientist of the data science platform Kaggle, where he was the top ranked participant in international machine learning competitions 2 years running. He was the founding CEO of two successful Australian startups (FastMail, and Optimal Decisions Group–purchased by Lexis-Nexis). Before that, he spent 8 years in management consulting, at McKinsey & Co, and AT Kearney. Jeremy has invested in, mentored, and advised many startups, and contributed to many open source projects.
He has many media appearances, including writing for the Guardian, USA Today, and the Washington Post, appearing on ABC (Good Morning America), MSNBC (Joy Reid), CNN, Fox News, BBC, and was a regular guest on Australia’s highest-rated breakfast news program. His talk on TED.com, “The wonderful and terrifying implications of computers that can learn”, has over 2.5 million views. He is a co-founder of the global Masks4All movement.
Submission of Papers
We invite three types of submissions for AusDM’21:
- Research Track: Academic submissions reporting on new algorithms, novel approaches and research progress, with a paper length of between 8 and 15 pages in Springer CCIS style, as detailed below.
- Application Track: Submissions reporting on applications of data mining and machine learning and describing specific data mining implementations and experiences in the real world. Submissions in this category can be between 6 and 15 pages in Springer CCIS style, as detailed below.
- Industry Showcase Track: Submissions from governments and industry on an analytics solution that has raised profits, reduced costs and/or achieved other important policy and/or business outcomes can be made in this track. Submissions to this category should be a 1-page extended abstract. Note that this track is presentation only, without publication in conference proceedings. For publication of your papers, please submit them to the above Application Track.
All submissions, except for the Industry Showcase Track, will go through a double-blind review process, i.e. paper submissions must NOT include authors names or affiliations or acknowledgments referring to funding bodies. Self-citing references should also be removed from the submitted papers for the double-blinded reviewing purpose. The information can be added in the accepted final camera-ready submissions.

All submissions are required to follow the format specified for papers in the Springer Communications in Computer and Information Science (CCIS) style. Authors should consult Springer’s authors’ guidelines and use the proceeding templates, either in LaTeX or Word, for the preparation of their papers. The electronic submission must be in PDF only and made through the AusDM’21 Submission page. Springer encourages authors to include their ORCIDs in their papers. In addition, the corresponding author of each paper, acting on behalf of all the authors of the paper, must complete and sign a Consent to Publish form, through which the copyright for their paper is transferred to Springer.
If the Springer’s authors’ guidelines or proceeding templates (LaTeX or Word) links are not opening on your browser, you can get them from here.
Submitted papers must not be previously published or accepted for publication anywhere. They must not be submitted to any other conference or journal during the review process of AusDM’21.
Submit your paper through EasyChair at here.
Important Dates
- Paper submissions:
24 August7 Sept 2021 - Notification: 30 September 2021
- Camera-ready: 17 October 2021
- Conference: 13-15 December 2021
Organising Committee
Conference Chairs
• Richi Nayak, Queensland University of Technology
• Yanchang Zhao, Data61, CSIRO
• Graham William, The Australian National University
Program Chairs
• Yue Xu, Queensland University of Technology
• Rosalind Wang, Western Sydney University
• Anton Lord, Leap In!
Organising Chairs
• Khanh Luong, Queensland University of Technology
• Thirunavukarasu Balasubramaniam, Queensland University of Technology
Publicity Chair
• Md Abul Bashar, Queensland University of Technology
Publication Chair
• Yee Ling Boo, RMIT University
Industry Track Program Chairs
• Annette Slunjski, IAPA
• Warwick Graco, ATO
Steering Committee
• Simeon Simoff (Chair), University of Western Sydney
• Graham William (Chair), Australian National University
• Peter Christen, Australian National University
• Ling Chen, University of Technology Sydney
• Zahid Islam, Charles Sturt University
• Paul Kennedy, University of Technology Sydney
• Yun Sing Koh, The University of Auckland
• Jiuyong (John) Li, University of South Australia
• Richi Nayak, Queensland University of Technology
• Kok–Leong Ong, RMIT University
• Dharmendra Sharma, University of Canberra
• Glenn Stone, Western Sydney University
• Yanchang Zhao, Data61, CSIRO
Further Information
AusDM’21 website: https://ausdm21.ausdm.org/
Contact the organisers of AusDM 2020 at ausdm21@ausdm.org
AusDM LinkedIn Group: https://www.linkedin.com/groups/4907891/